How faithful is text summarisation?
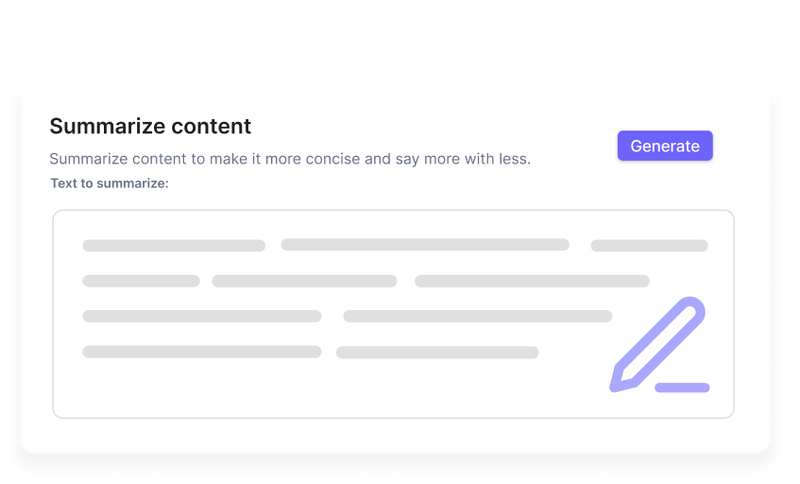
Jeremy Peckham - Research Lead at the AI, Faith and Civil Society Commission
What's this about?
GenAI and Large Language Models (LLMs) can be used for summarising reports, meeting notes, research papers and many more texts including book length works, provided the text is split into chunks. This requirement is due to the inability of most LLMs at this point to ingest text over a certain number of words.
"QuillBot's summarizer is trusted by millions worldwide to condense long articles, papers, or documents and into key summary paragraphs using state-of-the-art AI." [QuillBot website]
Measuring the faithfulness of GenAI text summarising
Researchers at the Allen Institute of AI and Princeton University, set out evaluate how faithful various LLMs were at summarising books. They chose books that were published after current LLMs had been trained to avoid data contamination. Human readers were used to assess how well each of the LLMs had summarised the books by evaluating claims, extracted from each of the summarised using ChatGPT, also an LLM based Gen AI system. The researchers called the process, Faithfulness Annotations in Book Length Summarisation (FABLES).
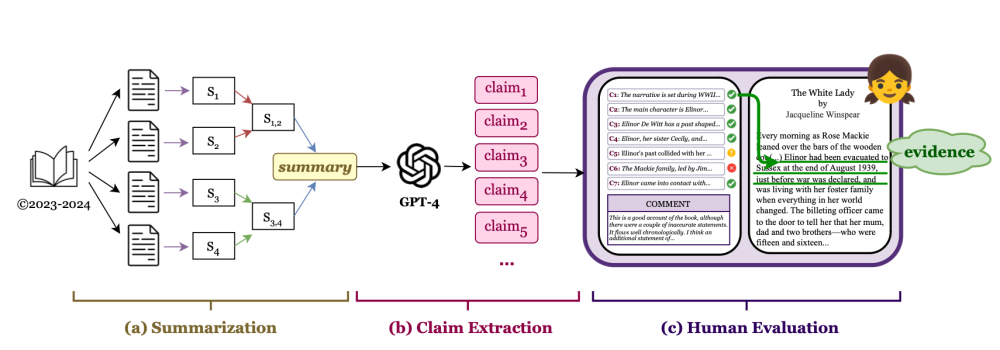
Pipeline for collecting faithfulness annotations in book-length summarization (FABLES).
SOURCE: Evaluating faithfulness and content selection in book-length summarization, Yekyung Kim metal, arXiv:2404.01261v1 [cs.CL] 1 Apr 2024
Extracting claims for evaluation
The longform summaries of the fiction books were decomposed into “atomic claims” for human readers to evaluate. The claims were produced automatically by prompting ChatGPT4 to produce that had to be fully understandable on their own and as far as possible, “situated within its relevant temporal, locational, and causal context”.

Example summary from the “Romantic Comedy”, by Curtis Sittenfeld, output by Claude 3 Opus. Adapted from Figure 2 in Yekyung Kim et al, April 2024.

Extracted claims output by ChatGPT4 from the example text summary produced by Claude 3 Opus. Claim numbers correspond to the annotated portions of the text summary shown above. Prompts were engineered for ChatGPT to ensure the claims were understandable and situated within the relevant text. Adapted from Figure 2 in Yekyung Kim et al, April 2024.
"Human validation by the authors of a random sample of 100extracted claims demonstrated 100% precision (i.e., each claim can be traced to the summarywithout any extra or incorrect information)." [Yekyung Kim et al - Allen Institute for AI & Princeton.]
How LLMs performed
Some LLMs did better than other with Claude 3 performing the best. None of them were completely faithful to the text of the books that they were summarising.
Faithfulness of various LLMs in summarising fiction books when evaluated by humans for accuracy of claims made that were extracted from the summaries by ChatGPT. Derived from data in Yekyung Kim et al, April 2024.
"A qualitative analysis of FABLES reveals that the majority of claims marked as unfaithful are related to events or states of characters and relationships. Furthermore, most of these claims can only be invalidated via multi-hop reasoning over the evidence, highlighting the task‘s complexity and its difference from existing fact-verification settings" Yekyung Kim et al - Allen Institute for AI & Princeton.
Types of error
The researchers at the Allen Institute for AI and Princeton University produced a taxonomy of errors. The percentage of summaries displaying each type of error levels are shown in the table below with omissions, factuality and chronology being the most problematic.
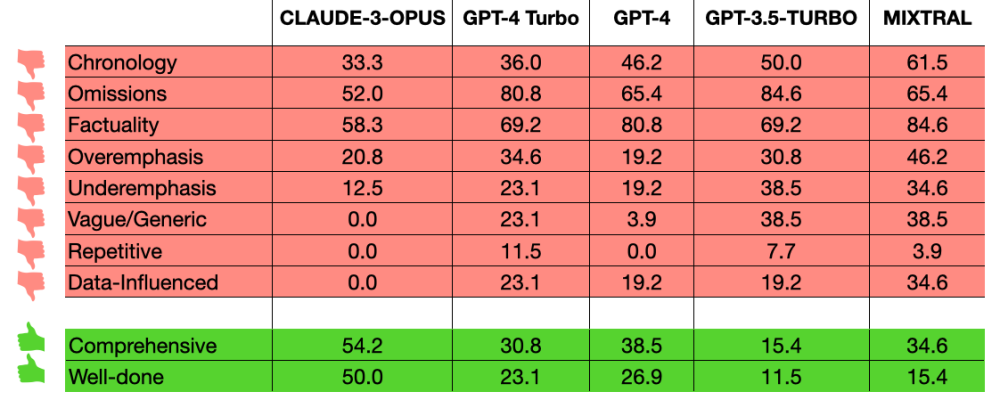
Percentage of summaries per model identified with specific issues shown in red boxes, based on annotator comments. The green boxes indicates categories where the models received compliments. Adapted from Table 6 Yekyung Kim et al, 2024.
"... omission of key information plagues all LLM summarizers." [Yekyung Kim et al - Allen Institute for AI & Princeton.]
Trustworthy or not?
This evaluation provides an important assessment of whether current GenAI systems can be trusted to produce a faithful summary of the texts that they have been fed. In particular it is alarming that such a high percentage of summaries were noted as having factual errors along with a not dissimilar percentage of omissions. This should cause us to pause and consider whether such systems should be relied upon at all in business, education and many other fields.
Human Values Risk Analysis
Truth & Reality: HIGH RISK
Significant percentage of Factual errors
Privacy & Freedom: HIGH RISK
LLMs use copyright data
Cognition & Creativity: MEDIUM RISK
Can reduce critical thinking
Dignity of Work: MEDIUM RISK
Replaces humans in producing summaries
Moral Autonomy: LOW RISK
No direct impact on moral autonomy
Authentic Relationships: LOW RISK
No direct impact on authentic relationships
Policy Recommendations
1. Organisations deploying a chatbot for use by the public or clients must be accountable for the output of the chatbot where there is consequential loss due to unfaithful summarisation of documents. Legislation may be needed to assign ‘product’ liability where the chatbot is the ‘product’.
2. Copyright protection should be enforced and no exception made for AI companies. Chapter 8 of the House of Lords Report cited, deals with Copyright in some detail and highlights various policy options and limitations of different approaches, such as licensing and opt in or opt out of data crawling on websites.
3. Developers and companies should be required to make information available on what data their system has been trained on and what accuracy they can be expected to achieve based on independent testing
References
Evaluating faithfulness and content selection in book-length summarization Yekyung Kim, Yapei Chang, Marzena Karpinska, Aparna Garimella , Varun Manjunatha , Kyle Lo, Tanya Goyalº, Mohit Iyyer UMass Amherst, Adobe , Allen Institute for AI, Princeton. April 2024