How has AI helped in the COVID pandemic?

Jeremy Peckham - Research Lead at the AI, Faith and Civil Society Commission
How has AI been used in the COVID-19 Pandemic?
AI researchers around the globe responded quickly to find ways to use machine learning and AI algorithms to help defeat the COVID-19 pandemic. Applications were developed in five key areas: Forecasting, Diagnosis, Containment and Monitoring, Drug Development and Treatments, and Medical and Social Management. Some, such as those in Forecasting, were aimed at predicting new outbreaks to assist policymaking, while others focused on reducing the impact on health services through remote triaging.
1. Forecasting
On December 31, BlueDot’s infectious disease risk assessment algorithm, which combines both human and artificial intelligence, alerted its customers to the outbreak in China—nine days before the WHO did. BlueDot uses foreign language news reports, animal and plant disease networks, as well as global ticketing data, to predict where and when infected individuals are likely to travel next. The algorithm does not rely on social media and accurately predicted that the virus would spread from Wuhan to Bangkok, Seoul, Taipei, and Tokyo within the following days (Bogoch, 2020).
Forecasting with AI is challenging because it requires large datasets to be effective, and there is no certainty that one virus will behave in the same way as another. Some attempts to use social media data to model the progress of COVID-19 have faced difficulties due to the noise in such data. For example, Google’s Flu Trends platform, which analysed search trends, overestimated doctor visits by more than double in 2014. This highlighted the conclusion that “big data” cannot replace traditional data collection and analysis, a phenomenon often referred to as “big data hubris.”
2. Diagnosis
The ability to diagnose and screen for COVID-19 is a critical part of managing the pandemic. Many AI based systems were developed to provide rapid diagnosis, even remotely for crude cough tests, using smart phones or an ordinary telephone. One of the challenges in developing remote diagnosis is how to deliver it to the whole population without discriminating against those that don’t have access to the required technology, such as a smart phone. These applications have not been scaled and have only been tested in small scale trials.
".... almost all published prediction models are poorly reported, and at high risk of bias such that their reported predictive performance is probably optimistic" [Laure Wynants, et al. BMJ 2020;369:m1328
One method of diagnosis relies on the use of image recognition to detect pneumonia like symptoms from X ray images and computerised tomography (CT) scans. Several literature surveys have been conducted to evaluate the reported results of these applications and they reach the same disappointing conclusions:
"Our review finds that none of the models identified are of potential clinical use due to methodological flaws and/or underlying biases." [Roberts, M., Driggs, D., Thorpe, M. et al. 2021]
3. Containment and Monitoring
Several approaches have been used to contain populations and monitor their movements or proximity to individuals known to be infected. Various proposals were made to ensure social distancing, such as using drones with facial recognition. Machine learning was also applied to predict the movement and spread of the virus, aiding in efforts to restrict movement and contain the outbreak. These strategies ranged from analysing travel data to monitoring social media.
In Asia, large-scale temperature checks were conducted in public places like train stations and building entrances, where individuals with elevated temperatures were denied access. Not all tracking and containment methods relied on AI, but China, for example, implemented a traffic light system using self-reported data and health records to control movement. A red light prohibited access, orange required quarantining, and green allowed free movement.
Various applications have been proposed for monitoring and controlling people's movement, such as drones, facial recognition, and GPS tracking. The most widely used method, which does not require AI, relies on smartphones to detect proximity to infected individuals through a track-and-trace system, using either GPS or less intrusive Bluetooth technology.

A schematic of app-based COVID-19 contact tracing (Fig. 4 from Ferretti et al. 2020). CC BY 4.0 via Wikimedia Commons.
The live threatening nature of the global pandemic as well as the threat to health care systems and the economy has been the main justification by governments for what, in normal times, would be regarded as an intrusion on privacy and freedom of movement and association.
"This is quite different from the traditional debate about whether confronting security threats to our way of life merits sacrificing the values of freedom and privacy that define us. Covid-19 is not an ideology, and rebalancing the contract between citizens and the state to take advantage of new technologies is not capitulation." [Chris Yiu, Tony Blair Institute for Global Change FT, 28 April 2020.]
Whether GPS or bluetooth based, concern has been expressed by civil liberties groups over the privacy of the data and the potential for wide scale tracking of people, perhaps even after a pandemic, once the apps have become established. A group of nearly 300 academics from around the world signed a “Joint Statement on Contact Tracing” to express their concerns about the adoption of the technology and to propose privacy principles for its use.
"It is vital that, in coming out of the current crisis, we do not create a tool that enables large scale data collection on the population, either now or at a later time." [Joint Statement on Contact Tracing 19 April 2020.]
It is interesting to note that there has not been a good take up of tracing apps around the globe, except in more authoritarian regimes where citizens can be forced to comply.
Although these applications did not require AI algorithms, it is to be expected that any centralised database will be used for Machine Learning to detect and predict patterns of behaviour and disease spread.
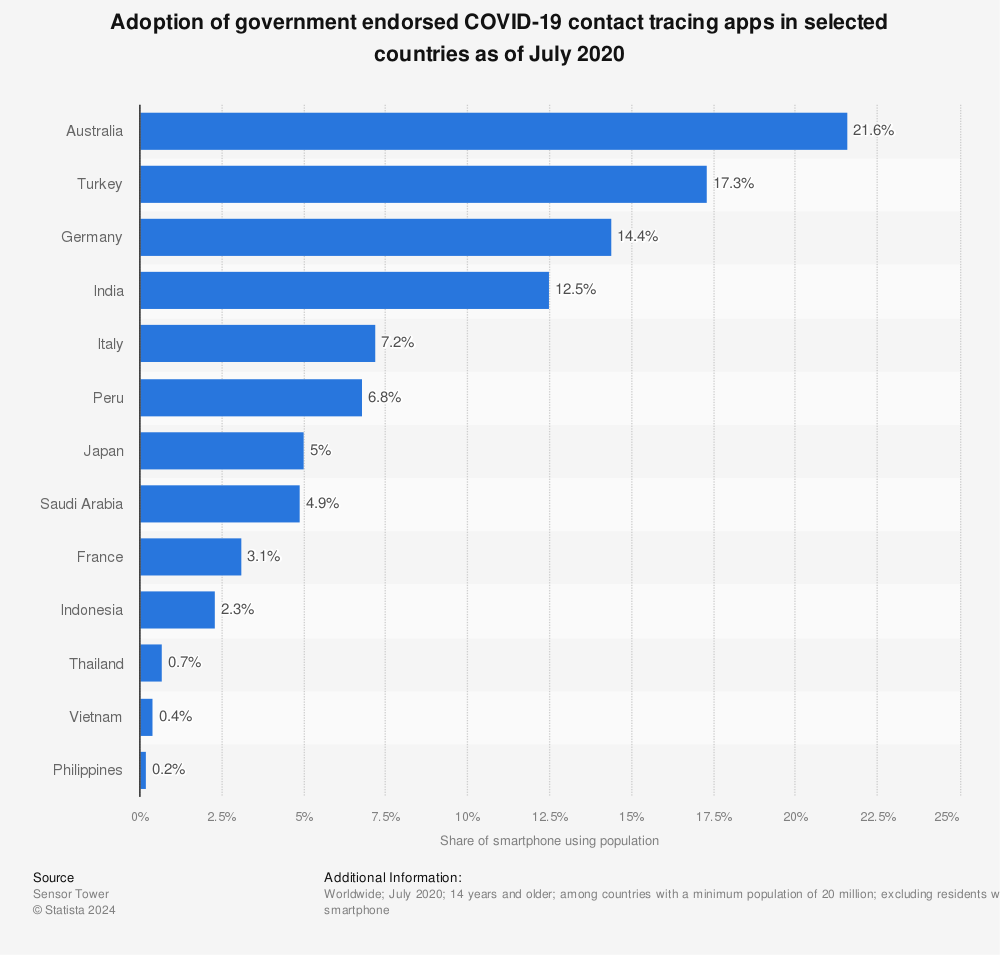
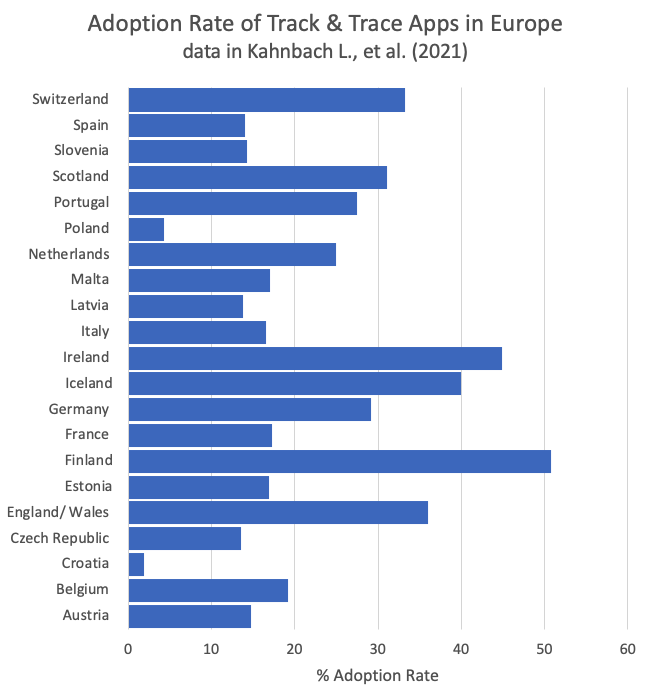
Find more statistics at Statista
In European the average adoption rate of contact tracing apps by the population was 22% with a large variation between countries such as Finland (51%) and Croatia (2%).
Between a 60% and 80% take up is regarded as being necessary to make such track and trace systems effective. The study in Europe did however report lower cumulative infection rates where there was a higher, but not ideal adaption rate of the governments track and trace application.
"Higher adoption rates were associated with lower cumulative infection rates. " [Kahnbach L., et al. 2021]
4. Drug Development and Treatments
Drug development is a long and extremely expensive process, taking on average 10 years and requiring a series of medical trials to evaluate the effectiveness and side effects of any proposed new treatment. Only around 1 in 1000 possible drug treatments progress from preclinical testing to full clinical trials and of those only 1 in ten make it to the approval stage.
AI algorithms are used in the early stages of drug development to help to reduce the initial number of compounds considered, including predicting likely adverse reactions.
"There are deep worries that a drug could prove ineffective or worse, dangerous, when used on populations that have a different response to the biomarker that was used to develop or validate that drug." [Ramamoorthy et al., 2015]
One of the success areas in the use of Machine Learning algorithms has been in the repurposing of drugs already approved for use in a particular condition. An example of this is the repurposing of a drug used in treating rheumatoid arthritis to alleviate the severe symptoms that can occur in some patients with COVID 19. The usefulness of AI in pattern matching as well as in discovering patterns, or connections, that are difficult for humans to spot easily, make it a powerful tool in learning from and searching millions of published texts and trials data.
In vaccine development, AI is being used along with systems biology to screen candidates and predict immune system response, through simulation, significantly cutting down the time normally taken in the development of a new vaccine. Very high levels of safety are required in vaccine development as they are being given to a very large population of healthy patients. It normally takes many years of rigorous development and testing of different vaccine candidates on animals, and then humans, before a safe and effective vaccine is licensed.
Machine Learning has also been used for taxonomic and hierarchical classification of COVID strains. Google owned DeepMind have used their deep learning algorithms in a program called Alphfold, to identify protein structure linked to COVID 19 that might be valuable for vaccine formulation.
5. Medical and Social Management
The Allen Institute for AI, in partnership with the CORD 19 Open Research Dataset, used its Natural Language Processing tools to allow academics and the general public to search publications related to COVID 19. Microsoft, also a CORD 19 partner, has created a COVID 19 data lake, providing researchers access to various data sets related to the pandemic.
Aimed at the general public, Natural Language based Chatbots have been deployed by many public health authorities around the world, including the World Health Organisation and the Centre for Disease Control, to provide health advice and information about the COVID 19.pandemic.
Limitations of AI
Whilst AI has been and continues to be useful in some areas of managing the COVID 19 pandemic, such as drug repurposing and forecasting infection spread, there are clear limitations to its use in a clinical setting.
"While AI has been practically applied for the identification of candidate drugs for drug repurposing and contact tracing , its application and utility for COVID-19 in clinical settings have been insignificant to date." [Chee, M.L., et al. 2021]
"AI has not yet been impactful against COVID-19. Its use is hampered by a lack of data, and by too much data (noisy and outlier). Overcoming these constraints will require a careful balance between data privacy and public health, and rigorous human-AI interaction. It is unlikely that these will be addressed in time to be of much help during the present pandemic." [Naude, W.2020]
"Problems around data availability, access and standardisation spanned the entire spectrum of data science activity during the pandemic. .... better data would enable a better response.... issues of inequality and exclusion related to data science and AI arose during the pandemic. These included concerns about inadequate representation of minority groups in data, and low engagement with these groups, which could bias research and policy decisions." [Alan Turing Institute, 2021]
Human Values Risk Analysis
1. Privacy & Freedom: HIGH RISK
AI-driven monitoring (e.g., contact tracing, facial recognition) raises significant privacy concerns, especially with potential post-pandemic surveillance.
2. Truth & Reality: MEDIUM RISK
AI forecasting and diagnosis have limitations, with inaccuracies and biases affecting truthfulness
3. Moral Autonomy: MEDIUM RISK
AI monitoring and health protocols may limit personal freedom, and biases in AI could influence moral decision-making.
4. Dignity of Work: MEDIUM RISK
AI aids efficiency but could displace workers or reduce human involvement in healthcare, affecting job dignity.
5. Authentic Relationships: LOW RISK
No direct impact on authentic relationships
6. Cognition and Creativity: LOW RISK
No direct impact on cognition and creativity
Policy Recommendations
1. Data Privacy and Security
Establish strict privacy protections for AI-driven health apps and ensure consent protocols are clear and respected.
2. Standardise Data Collection and Reporting
Implement standard frameworks for transparent data collection, reporting, and AI model validation to improve reliability.
3. Address Bias and Inequality
Fund research to reduce AI bias and ensure diverse representation in health data to avoid discrimination in healthcare applications.
4. AI Transparency and Accountability
Require developers to disclose AI algorithms’ data sources, training methods, and potential risks, ensuring accountability for outcomes.
5. International Collaboration
Promote international data sharing and collaboration to address global health crises effectively and equitably.
References
Bogoch II, Watts A, Thomas-Bachli A, Huber C, Kraemer MUG, Khan K. Pneumonia of unknown aetiology in Wuhan, China: potential for international spread via commercial air travel. J Travel Med. 2020 Mar 13;27(2):taaa008. doi: 10.1093/jtm/taaa008. PMID: 31943059; PMCID: PMC7107534.
Chee ML, Ong MEH, Siddiqui FJ, Zhang Z, Lim SL, Ho AFW, Liu N. Artificial Intelligence Applications for COVID-19 in Intensive Care and Emergency Settings: A Systematic Review. Int J Environ Res Public Health. 2021 Apr 29;18(9):4749. doi: 10.3390/ijerph18094749. PMID: 33947006; PMCID: PMC8125462.
Data science and AI in the age of COVID-19: Reflections on the response of the UK’s data science and AI community to the COVID-19 pandemic, Alan Turing Institute, 2021.
Kahnbach L, Lehr D, Brandenburger J, Mallwitz T, Jent S, Hannibal S, Funk B, Janneck M
Quality and Adoption of COVID-19 Tracing Apps and Recommendations for Development: Systematic Interdisciplinary Review of European Apps
J Med Internet Res 2021;23(6):e27989
Track & Trace Diagram from Ferretti, Luca; Wymant, Chris; Kendall, Michelle; Zhao, Lele; Nurtay, Anel; Abeler-Dörner, Lucie; Parker, Michael; Bonsall, David; Fraser, Christophe (2020-03-31). “Quantifying SARS-CoV-2 transmission suggests epidemic control with digital contact tracing” CC BY 4.0
Naudé, W. Artificial intelligence vs COVID-19: limitations, constraints and pitfalls. AI & Soc 35, 761–765 (2020). https://doi.org/10.1007/s00146-020-00978-0
Patrick McGee and Hannah Murphy, Financial Times, April 28 2020, Coronavirus apps: the risk of slipping into a surveillance state, https://www.ft.com/content/d2609e26-8875-11ea-a01c-a28a3e3fbd33
Prediction models for diagnosis and prognosis of covid-19: systematic review and critical appraisal, BMJ 2020; 369 doi: https://doi.org/10.1136/bmj.m1328 (Published 07 April 2020) BMJ 2020;369:m1328 (CC BY 4.0)
Ramamoorthy, A., Pacanowski, M. A., Bull, J., & Zhang, L. (2015). Racial/ethnic differences in drug disposition and response: review of recently approved drugs. Clinical Pharmacology & Therapeutics, 97(3), 263-273.
Roberts, M., Driggs, D., Thorpe, M. et al. Common pitfalls and recommendations for using machine learning to detect and prognosticate for COVID-19 using chest radiographs and CT scans. Nat Mach Intell 3, 199–217 (2021). https://doi.org/10.1038/s42256-021-00307-0
Šćepanović, S., Aiello, L. M., Zhou, K., Joglekar, S., & Quercia, D. (2021). The Healthy States of America: Creating a Health Taxonomy with Social Media. Proceedings of the International AAAI Conference on Web and Social Media, 15(1), 621-632. Retrieved from https://ojs.aaai.org/index.php/ICWSM/article/view/18089